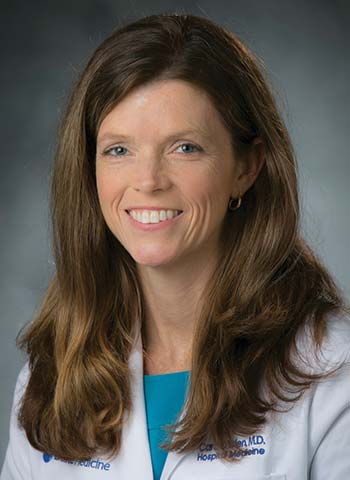
With sepsis, time is of the essence. Left untreated, the illness—a runaway immune response to infection—can quickly become life-threatening. Hours, and even minutes, can mean the difference between life and death for this condition, which strikes more than 1.5 million people and kills more than 250,000 Americans each year.
Because sepsis is nonspecific, there is no single definitive sign, symptom, or test that identifies a patient as having it. That’s a huge challenge for providers, who need to assess patients as quickly as possible.
Duke hospital medicine physician Cara O’Brien, MD, is partnering with the Duke Institute for Health Innovation to lead a multidisciplinary team of physicians, nurses, and biostatisticians that is using data science to rapidly identify at-risk patients, speed treatment, and improve outcomes.
“We have a tremendous data source in the electronic health record—vital signs, labs, co-morbidities, nursing assessments—that we can harvest to predict various disease states,” she explains.
O’Brien and her team culled over 32 million data elements from patients in Duke’s electronic health record (EHR) system to identify key variables as potential predictors for sepsis. They created a predictive model and tested it on retrospective patient data, where they found that the model identified sepsis about five hours earlier than other models—a substantial lead time that would allow clinicians to start treatment much sooner. In November, they launched a real-time, prospective trial in the Emergency Department (ED) at Duke Hospital.
“Eighty percent of sepsis cases develop within the first six hours of a patient’s hospital stay, likely either present at the time of arrival or developed soon after,” O’Brien says. “That’s why we chose to deploy our model in the ED first.”
Any time an ED patient’s EHR is updated, whether for vitals, labs, or assessments, that data is immediately fed through the model, which generates a risk score at that moment. A team of nurses monitors those risk profiles and alerts the ED staff of any high-risk patients so they can monitor and begin treatment quickly if sepsis appears.
Duke is the first health system in the country to implement a machine learning predictive model in real time to predict sepsis. If this trial is successful, this could be transformative for treatment of sepsis nationally and globally.
This story was originally published in the Spring 2019 issue of DukeMed Alumni News
By Laura Ertel
Read more about Big Data in Action
- Forging the Future
- Fine-tuning Treatments for Breast Cancer
- Training Computers to Improve Cardiovascular Care